Introduction
Asymmetric synthesis is a critical area of chemistry that relies heavily on the precise control of molecular chirality. While experimental methods have traditionally driven advancements in this field, the integration of computational chemistry has significantly enhanced our understanding and capabilities. Computational approaches offer several advantages, including the ability to model complex systems, predict reaction outcomes, and optimize catalysts. This blog explores the role of computational chemistry in asymmetric synthesis, showcasing its techniques, applications, and future potential.
Asymmetric Catalysis
Asymmetric catalysis has played a pivotal role in transforming the field of organic synthesis over the past fifty years. This revolutionary approach focuses on creating stereogenic centers, which are crucial for the formation of new carbon-carbon bonds and the reduction of unsaturated bonds such as carbonyls and alkenes. The innovation of chiral catalysts has been central to these advancements. These catalysts come in several forms, including transition metal complexes, organocatalysts, and biocatalysts, each offering unique advantages and applications.
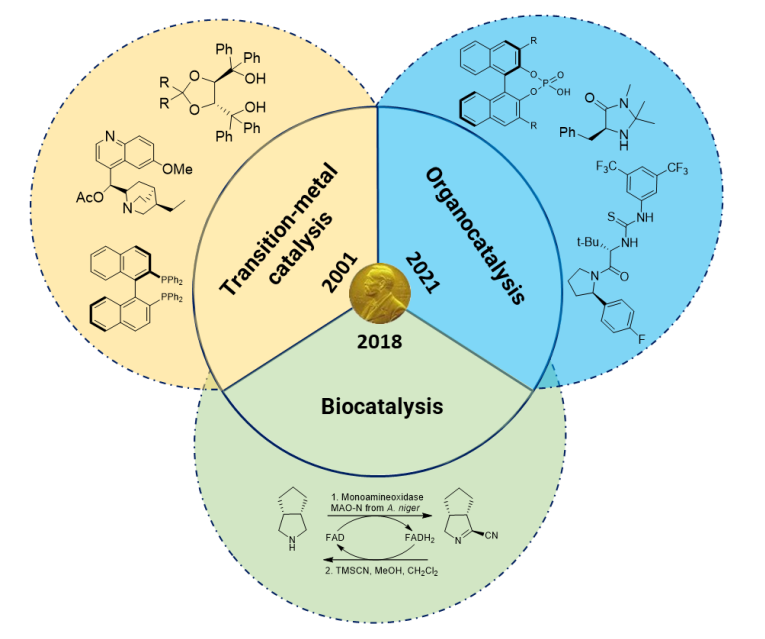
Main Asymmetric Catalysis Fields
Recognition through the award of Nobel Prizes in Chemistry
Transition metal complexes have traditionally been used in asymmetric catalysis, where the metal itself acts as the catalyst. The introduction of stereochemistry is achieved through metal ligands, such as chiral phosphines. These metal complexes have been highly effective in various reactions, particularly those involving the formation of new carbon-carbon bonds. However, the cost and toxicity associated with transition metal catalysts have driven the search for alternatives.
Organocatalysis and biocatalysis have emerged as promising alternatives to transition metal complexes. Organocatalysis utilizes small organic molecules as catalysts, which also serve as chiral directing groups. These small organic catalysts are advantageous due to their lower cost and reduced environmental impact compared to transition metal catalysts. Similarly, biocatalysis employs enzymes, which are nature’s catalysts, to achieve stereoselective reactions. Enzymes are highly specific and efficient, making them ideal for asymmetric catalysis. The development of these alternatives has been recognized by the scientific community, as evidenced by the Nobel Prizes awarded to Frances Arnold (2018) for her work on the directed evolution of enzymes, and to Benjamin List and David MacMillan (2021) for their contributions to asymmetric organocatalysis. These achievements build on the foundational work of Barry Sharpless, Ryoji Noyori, and William Knowles, who were awarded the Nobel Prize in 2001 in chemistry for their pioneering efforts in asymmetric transition metal catalysis.
Role of Computational Methods in Asymmetric Organocatalysis
The field of organocatalysis has undergone significant evolution since its inception in the 1990s. This evolution has been driven by the development of novel catalytic strategies that lay a strong foundation for the future of robust asymmetric organocatalytic scaffolds. While academic research in organocatalysis has advanced rapidly, industrial applications have lagged behind. However, recent efforts to investigate the recyclability and stability of immobilized organocatalysts are paving the way for broader industrial adoption.
Design approaches in organocatalysis have shifted from purely experimental methods to those that incorporate computational techniques. This shift emphasizes the importance of data-driven approaches. Machine learning (ML) has emerged as a particularly powerful tool for predicting molecular properties and catalytic reactions. The success of ML models depends heavily on the quality and quantity of available databases and the choice of molecular descriptors. As computational technology continues to advance, ML is poised to become an essential tool for designing better catalysts and optimizing reactions.

Evolution of Organocatalysis
Over the past decade, the field of asymmetric organocatalysis has significantly advanced. This progress is attributed to the development of key synthetic methods and the innovative integration of experimental and computational techniques. With the ongoing evolution of the field and the growth of computing technology, further breakthroughs in asymmetric organocatalysis are inevitable. Over the past ten years, we’ve seen some incredible strides in the field of asymmetric organocatalysis. This progress is thanks to the development of essential synthetic methods and the exciting blend of hands-on experiments with cutting-edge computational techniques. As the field continues to evolve and computing technology advances, we can look forward to even more groundbreaking discoveries in asymmetric organocatalysis.
Computational methods involves the use of computer simulations to solve chemical problems. In asymmetric synthesis, it plays a crucial role by providing insights into the mechanisms of chiral reactions, predicting the behavior of chiral catalysts, and guiding the design of new catalysts. The main advantages of computational approaches include:
- Efficiency: Computational methods can quickly screen a vast number of potential catalysts, significantly reducing the time and resources needed for experimental trials.
- Accuracy: High-level computational models can predict enantioselectivities and reaction outcomes with remarkable accuracy, guiding chemists in selecting the most promising catalysts.
- Insight: Detailed simulations provide a deep understanding of reaction mechanisms, helping to identify key factors that influence enantioselectivity.
Molecular Modeling and Simulation for Chiral Catalyst design
Docking-based virtual screening has become a widely accepted tool in medicinal chemistry for the design of new drugs. This approach involves simulating the interaction between a target molecule and potential drug candidates to predict binding affinities and identify promising compounds. The low computational demands and user-friendly interfaces of these methods have facilitated their widespread adoption. However, similar computational tools for chemical reaction development remain underutilized in the field of asymmetric catalysis. Quantum mechanics (QM) calculations, particularly density functional theory (DFT), are powerful tools for understanding reaction mechanisms and rationalizing observed selectivities. These methods provide detailed insights into the electronic structure of molecules and the transition states of reactions. However, QM calculations are typically used in a retrospective, post-hoc fashion rather than for predictive purposes. The high computational costs and required expertise associated with ab initio QM or DFT methods make them impractical for screening large libraries of potential catalysts. Despite these challenges, significant efforts are underway to develop computational tools that can assist organic chemists in the design of new catalysts. Integration of these tools into organic chemistry laboratories is imminent, driven by advances in computational power and the development of user-friendly software
Molecular modeling techniques, such as density functional theory (DFT) and molecular dynamics (MD) simulations, are commonly used to study chiral catalysts. DFT is particularly useful for calculating the electronic structure of molecules and predicting the energy barriers of chemical reactions. MD simulations, on the other hand, allow for the exploration of the dynamic behavior of molecules over time, providing insights into the flexibility and conformational changes of chiral catalysts.
Machine Learning – State-of-the-at-Approaches
The field of computer-assisted synthesis has gained substantial momentum in recent years, with several computational methods being applied to organic synthesis problems. Machine learning (ML) and Statistical models have been particularly effective in this regard. For example, computer-aided synthesis planning tools like Chematica/Synthia and AIZynthFinder have advanced rapidly. These tools can propose reaction pathways, predict yields, assess catalyst inhibition, and evaluate regioselectivity and chemical reactivity.
ML techniques offer significant speed advantages over traditional quantum mechanics (QM) and molecular mechanics (MM) methods, particularly for reactions with complex or unclear mechanisms. ML models can be trained to predict the stereochemical outcomes of asymmetric reactions based solely on the structures of the catalysts. This capability is particularly valuable for reactions where the mechanisms are not well understood. However, training ML models requires a substantial amount of experimental data, which can be a limiting factor for new or poorly studied reactions.
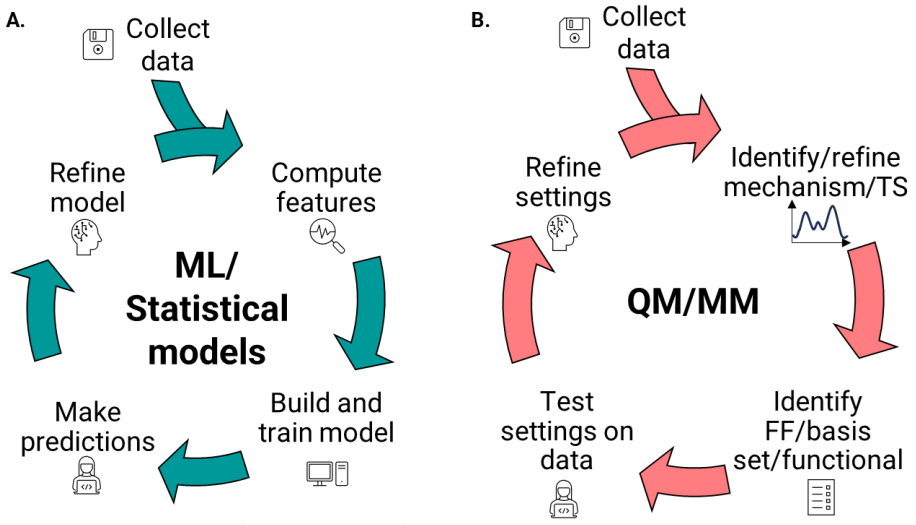
Overall workflow for A) ML methods and B) QM/MM methods.
In computational asymmetric catalysis, a key objective is to predict the enantioselectivity of symmetric reactions, which helps optimize reactions computationally by identifying the best catalysts, substrates, ligands, and conditions. There are two main approaches to achieve this: (1) methods that require a deep understanding of the reaction mechanism, such as quantum mechanics (QM) and molecular mechanics (MM) methods, and (2) data-driven methods, which rely on databases and machine learning (ML). Regardless of the approach, the process generally involves collecting data, identifying significant patterns or features, building and testing models, and then refining those models.
Predictive models are developed by combining computational techniques with experimental data. These models can forecast the outcomes of asymmetric reactions, such as the enantiomeric excess (ee) and yield, under various conditions. Machine learning algorithms are increasingly being employed to improve the accuracy of these predictions by identifying patterns in large datasets of reaction outcomes.
Virtual Screening of Catalysts
Virtual screening involves the computational evaluation of large libraries of potential catalysts to identify those with the highest potential for enantioselective reactions. This approach has been successfully applied to the discovery of new chiral organocatalysts and transition metal complexes. By simulating the interactions between catalysts and substrates, researchers can quickly narrow down the most promising candidates for experimental testing.
The development of computational tools to support organic synthesis, including the prediction of reaction pathways, optimization, and selectivity, is a significant area of interest. Transition state force fields (TSFFs), derived from quantum-guided molecular mechanics, Q2MM, methods, enable rapid and accurate stereoselectivity predictions for organic reactions. These TSFFs are developed by fitting the parameters of a force field, such as MM3* or AMBER, to the results of electronic structure calculations ( (Image below, left). CatVS is an automated tool for the virtual screening of substrate and ligand libraries for asymmetric catalysis. It facilitates the screening process within hours, significantly enhancing the efficiency of high-throughput experimentation. For example, in the OsO4-catalyzed cis-dihydroxylation, the results from CatVS’s automated setup are indistinguishable from those obtained through manual substrate screening. Similarly, predictive computational ligand selection has been demonstrated in the virtual screening of diphosphine ligands for the rhodium-catalyzed asymmetric hydrogenation of enamides. Experimental testing has verified that the most selective substrate-ligand combinations identified by CatVS are indeed the most effective, underscoring the tool’s potential to streamline the catalyst development process. Image below presents the flow chart for the combined Q2MM–CatVS method.
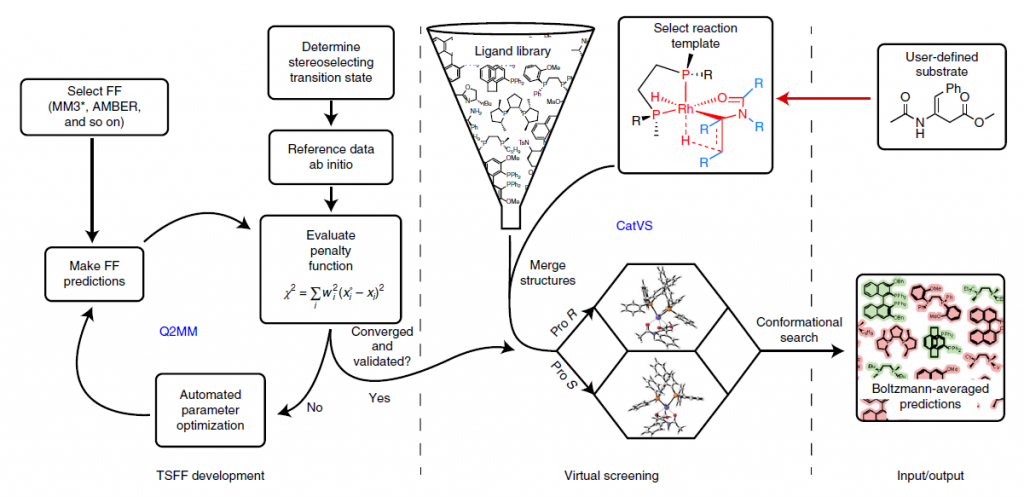
Flowchart for CatVS
User-selected substrates are combined with ligands from a predefined library on a reaction template. This is followed by an automated conformational search using TSFF parameters and Boltzmann averaging of the free energies for the ensembles of diastereomeric transition states. FF stands for force field.
Integration with Experimental Methods
Complementary Role of Computation and Experimentation
Computational and experimental methods complement each other in asymmetric synthesis. While computational models provide predictions and insights, experimental validation is essential to confirm these findings. This iterative process—where computational predictions guide experiments and experimental results refine computational models—accelerates the development of effective chiral catalysts.
Enhancing Catalyst Performance Through Iterative Processes
Iterative processes involving computation and experimentation enable the fine-tuning of catalyst performance. For instance, initial computational screening can identify promising catalysts, which are then synthesized and tested experimentally. The experimental data can be fed back into computational models to further refine the catalyst design. This cycle continues until the desired level of enantioselectivity and efficiency is achieved.
Future Directions
AI and Machine Learning in Catalyst Design
Machine learning (ML), a prominent strategy within artificial intelligence (AI), leverages algorithms to learn from data, detect patterns, and make accurate predictions. ML is an interdisciplinary field combining computer science, statistics, and data science. It focuses on automated learning processes that improve over time through decision-making under uncertainty.
Supervised learning, one of the most widely used ML methods, has driven much of the practical success in deep learning. This approach involves training a model on a labeled dataset, where the desired output is known. Various supervised learning algorithms, including linear regression, artificial neural networks (ANNs), support vector machines (SVMs), random forests, and decision trees, are employed to solve different tasks.
Development of suitable machine-learning (ML) approaches by using molecular descriptors can provide significant impetus to current efforts in asymmetric catalysis, wherein one strives to make a desired stereoisomer of a given handedness (enantiomer) in a highly selective manner. The proposed approach provides a sustainable model that trains on known catalysts and helps to predict the efficacy of additional catalysts for asymmetric synthesis, thereby expediting the discovery with lesser cost as compared to traditional empirical methods. Training ML algorithms first using the available known examples -predicting additional catalysts using such algorithms for subsequent in-line experimental validation, re-training by augmenting with the additional data thus generated – can provide a superior train–predict–train cycle suitable for accelerated reaction discovery.
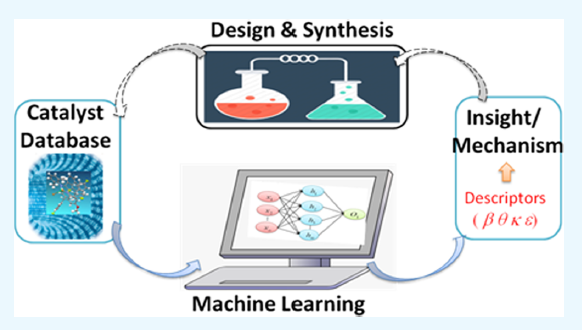
ML technique toward design and development
(in the area of homogeneous and heterogeneous catalysis)
Despite ML’s growing popularity and predictive power in various academic and industrial applications, its application in catalysis remains relatively underdeveloped. Modern statistical learning techniques hold strong potential for optimizing and discovering catalysts. By converting available data into practical, useful models, these techniques can uncover underlying mechanisms and predict the behavior of catalysts in new reactions. Current applications in homogeneous and heterogeneous catalysis demonstrate the potential for ML to revolutionize the field, making it possible to design more efficient and selective catalysts.
Automated Asymmetric Synthesis
The development of new methods that incorporate ML techniques is an ongoing process. One innovative approach involves data-driven automation laboratories. These labs utilize robotic synthesis engines ‘Chemspeed’ and ML to automate the entire synthetic workflow. The integration of robots and ML enables the collection of high-quality experimental data, which can then be used to iteratively improve ML models.
Despite their promise, automated labs face challenges in integrating human cognitive processes and addressing unexpected results. For example, these labs may struggle with integrating human intuition, dealing with unexpected or difficult-to-predict outcomes, and identifying unknown or unexpected products and side products. Additionally, the software used in these labs is often designed without self-driving laboratories in mind, which can limit their effectiveness.
Currently, the applications of automated labs are primarily limited to organometallic chemistry. However, there is significant potential for applying these technologies to asymmetric organocatalysis. The combination of automated labs with advancements in continuous flow systems could greatly enhance the field of organic asymmetric catalysis. By automating the synthesis process, these labs could significantly accelerate the development of new catalysts and reaction conditions.

Diagrammatic depiction of the autonomous laboratories
The self-driven lab uses a highly flexible and versatile software package, ‘ChemOs’, which orchestrates experiment scheduling and selects future experiments using feedback from previous experiments through ML. In addition to providing highly reproducible results by eliminating human error, these systems provide higher throughput, collecting large amounts of quantitative data in comparison to ML models alone. The development of such systems can potentially expedite molecular discovery significantly by enabling systematic optimizations, increasing efficiency, and decreasing the consumption of materials.
Robots and A.I. team up
Artificial intelligence (A.I.) has made headlines recently with the advent of ChatGPT’s language processing capabilities. Researchers are developing ML methods to predict highly selective asymmetric catalysts using advanced 2D chemical descriptors. These descriptors represent molecular structures in a way that is computationally efficient, eliminating the need for extensive quantum chemical computations. The predictive model can be run on standard laptops, making it accessible to a wide range of researchers.
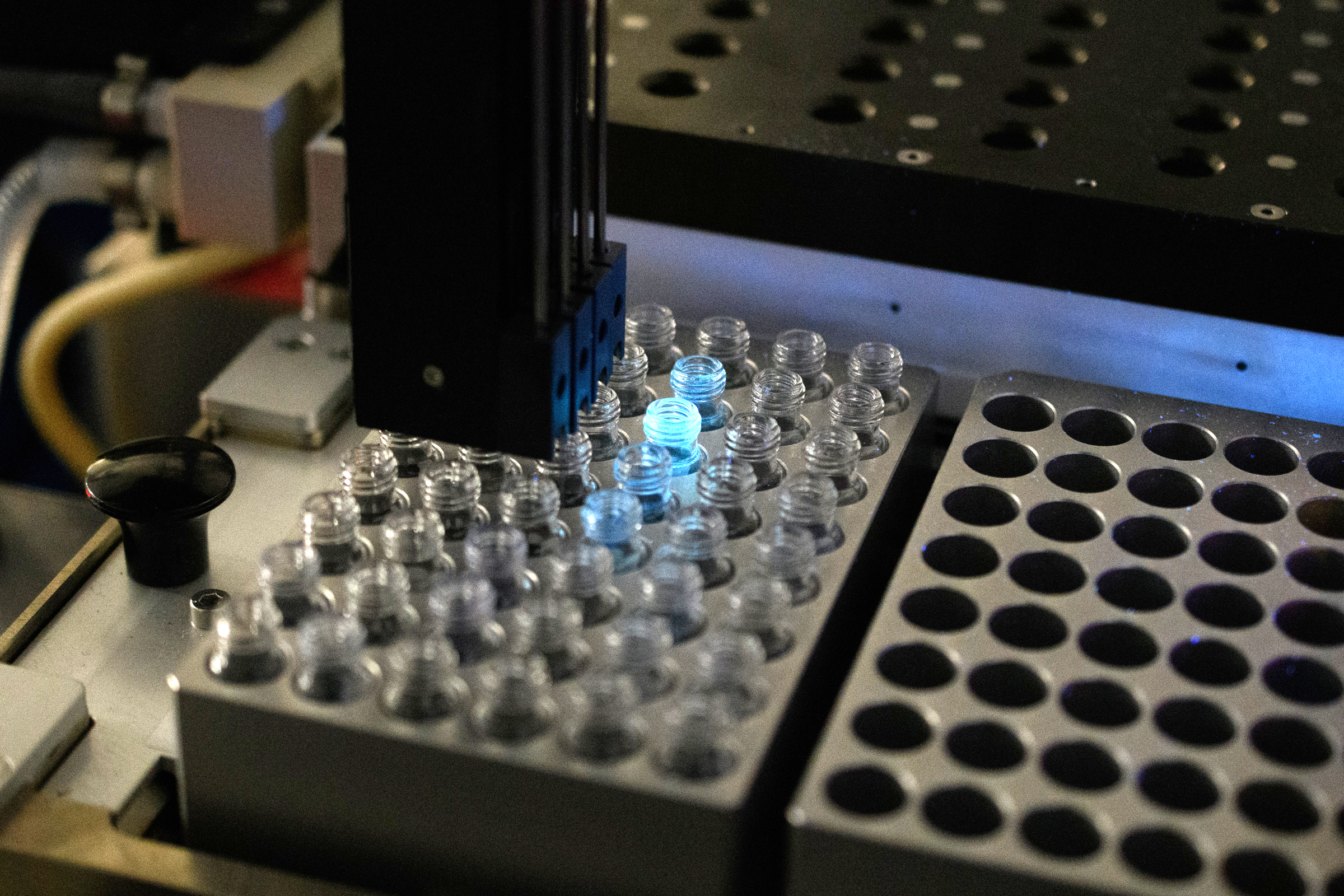
Source: https://www.global.hokudai.ac.jp/blog/robots-and-a-i-team-up-to-discover-highly-selective-catalysts/
Semi-automated synthesis robot used to generate training data
For a computer to learn chemical information, molecules are often represented using descriptors, which are essentially fragments or small parts of those molecules. These fragments are easier for A.I. to process and can be arranged in various ways to form different molecules, similar to how Lego pieces can be connected to create different structures. However, 2D descriptors, which are computationally less intensive, have had difficulty accurately representing complex catalyst structures, leading to unreliable predictions. To address this, researchers developed new Circular Substructure (CircuS) 2D descriptors that specifically account for cyclic and branched hydrocarbon structures, which are common in catalysis. The training data for the A.I. was obtained through experiments using a streamlined, semi-automated process with a synthesis robot. This experimental data was then transformed into descriptors and used to train the A.I. model.
Potential Breakthroughs
The integration of AI and ML with computational chemistry could lead to several breakthroughs, such as:
- The discovery of entirely new classes of chiral catalysts with unprecedented selectivity and efficiency.
- Up the discovery process.
- Enhanced understanding of complex catalytic mechanisms, enabling the rational design of next-generation catalysts.
The development of automated platforms for catalyst design and optimization, significantly speeding Artificial intelligence (AI) and machine learning (ML) are poised to revolutionize catalyst design in asymmetric synthesis. By training ML algorithms on large datasets of catalytic reactions, researchers can develop models that predict the performance of new catalysts with high accuracy. AI can also assist in the automated discovery of novel catalysts by exploring vast chemical spaces that are beyond the reach of traditional methods.
Conclusion
Computational methods have become indispensable tools in the field of asymmetric synthesis, offering numerous benefits such as efficiency, accuracy, and deep mechanistic insights. The complementary use of computational and experimental approaches has already led to significant advancements in chiral catalyst design and reaction optimization. As AI and machine learning continue to evolve, they promise to further enhance the capabilities of computational chemistry, leading to new breakthroughs in asymmetric synthesis. Encouraging interdisciplinary collaboration between computational chemists, experimentalists, and data scientists will be key to unlocking the full potential of these technologies, driving the future of chiral chemistry forward. Understanding and harnessing computational methods in asymmetric synthesis not only accelerates research but also opens up new avenues for innovation, ultimately contributing to the development of more effective and sustainable chemical processes.
Further Reading
Pinus S, Genzling J, Burai-Patrascu M, Moitessier N. Catalyzing Change: The Power of Computational Asymmetric Catalysis. ChemRxiv. 2023; doi:10.26434/chemrxiv-2023-t29k7. DOI: 10.26434/chemrxiv-2023-t29k7
Nika Melnyk, et al., Evolution of design approaches in asymmetric organocatalysis over the last decade. Tetrahedron Chem, 2023. https://doi.org/10.1016/j.tchem.2023.100035
Rosales, A.R., Wahlers, J., Limé, E. et al. Rapid virtual screening of enantioselective catalysts using CatVS. Nat Catal. 2019, 2, 41–45. https://doi.org/10.1038/s41929-018-0193-3
Reid, J.P., Sigman, M.S. Holistic prediction of enantioselectivity in asymmetric catalysis. Nature, 2019, 571, 343–348. https://doi.org/10.1038/s41586-019-1384-z
React. Chem. Eng., 2021, 6, 694-708.
Digital Discovery, 2022, 1, 926-940.
Ben Gao, Liu Cai, Yuchen Zhang, Huaihai Huang, Yao Li & Xiao-Song Xue, A Machine Learning Model for Predicting Enantioselectivity in Hypervalent Iodine(III) Catalyzed Asymmetric Phenolic Dearomatizations, CCS Chem. 2024, DOI: 10.31635/ccschem.024.202303774.
Dr. Nobuya Tsuji, Dr. Pavel Sidorov, Dr. Chendan Zhu, Dr. Yuuya Nagata, Dr. Timur Gimadiev, Prof. Dr. Alexandre Varnek, Prof. Dr. Benjamin List, Predicting Highly Enantioselective Catalysts Using Tunable Fragment Descriptors, 2023. https://doi.org/10.1002/anie.202218659
Yulong Chen, et al., Iterative Approach of Experiment–Machine Learning for Efficient Optimization of Environmental Catalysts: An Example of NOx Selective Reduction Catalysts, Environmental Science & Technology, 2023, 57, 18080-18090. doi: 10.1021/acs.est.3c00293
Singh S, Pareek M, Changotra A, Banerjee S, Bhaskararao B, Balamurugan P, Sunoj RB. A unified machine-learning protocol for asymmetric catalysis as a proof of concept demonstration using asymmetric hydrogenation. Proc Natl Acad Sci U S A. 2020; 117(3):1339-1345. doi: 10.1073/pnas.1916392117.
Karthikeyan, A., Priyakumar, U.D. Artificial intelligence: machine learning for chemical sciences. J Chem Sci. 2022, 134, 2. https://doi.org/10.1007/s12039-021-01995-2.
N. Tsuji, P. Sidorov, C. Zhu, Y. Nagata, T. Gimadiev, A. Varnek, B. List, Angew. Chem. Int. Ed. 2023, 62, e202218659; Angew. Chem. 2023, 135, e202218659.
Rosales, A. R., Wahlers, J., Limé, E., Meadows, R. E., Leslie, K. W., Savin, R., Norrby, P.-O. Rapid virtual screening of enantioselective catalysts using CatVS. Nature Catalysis, 2018. doi:10.1038/s41929-018-0193-3
Bell, E.L., Finnigan, W., France, S.P. et al. Biocatalysis. Nature Reviews Methods Primers , 2021, 1, 46. https://doi.org/10.1038/s43586-021-00044-z
Pyser, J. B., Chakrabarty, S., Romero, E. O., & Narayan, A. R. H. State-of-the-Art Biocatalysis. ACS Central Science, 2021, 7, 1105–1116. doi:10.1021/acscentsci.1c00273
Nobuya Tsuji, Pavel Sidorov, Chendan Zhu, Yuuya Nagata, Timur Gimadiev, Alexandre Varnek, Benjamin List. Predicting Highly Enantioselective Catalysts Using Tunable Fragment Descriptors. Angewandte Chemie International Edition.2023. DOI: 10.1002/anie.202218659.
Very insightful!
The role of computational chemistry in predicting the reaction outcome is an emerging scope. During our research on the challenging asymmetric Diels-Alder reactions between nitrostyrenes and cyclopentadiene, it was necessary to understand the mechanism of the reaction, as it generates two products (the usual Diels-Alder product, and also the Hetero Diels-Alder product). We applied Q2MM method to optimise the transition state.
The study wasn’t successful, however, the investigation did give us the answer to why out of 2 exo and 2 endo isomers, 1 is formed as major.
Thank you for sharing your insights! Computational chemistry is indeed vital for understanding reaction mechanisms. Your use of the Q2MM method in asymmetric Diels-Alder reactions is intriguing, even if the results weren’t as expected. It’s valuable that you discovered why one isomer predominates.
Dear Sir,
It is a well-crafted article that captures the transformative impact of computational methods in asymmetric synthesis. The depth of coverage, from traditional transition metal catalysis to the integration of AI and ML, reflects a thorough understanding of the field’s evolution. The logical flow from foundational concepts to advanced applications, and the distinction between traditional and modern approaches, make the article accessible to a broad audience. Your discussion on the role of ML in catalyst design, particularly using descriptors to train AI models, provides valuable insights. The exploration of docking-based virtual screening and QM/MM methods enriches understanding. The potential discoveries of new chiral catalysts underscore the importance of ongoing research at the intersection of AI, ML, and chemistry. The visual aids, such as flowcharts and diagrams, enhance the understanding of complex concepts and reinforce the text. The discussion on AI and ML’s future role in catalyst design is compelling, making the article a critical reference for future research. This article is a significant contribution to the field of asymmetric synthesis.
Thank You Sir
Thank you for your thoughtful feedback! I’m glad you found the article’s logical flow and distinction between traditional and modern approaches accessible. Your insights on the role of ML in catalyst design and the use of visual aids are much appreciated. The discussion on AI and ML’s future role in catalyst design is indeed exciting and promising for future research.
Dear Sir,
Your work brilliantly highlights the transformative impact of computational methods in asymmetric synthesis. The depth of insight and clarity in presenting the intersection of AI, machine learning, and chemistry is commendable. Your emphasis on interdisciplinary collaboration is both timely and inspiring, pointing the way to future innovations. Thank you for your valuable contributions to the field!
Thank you for your generous feedback! I’m thrilled that the intersection of computational methods, AI, and chemistry resonated with you. Indeed, interdisciplinary collaboration is our compass toward groundbreaking innovations in asymmetric synthesis.
Well compiled to give a clear understanding of the past, present and future trends of this field. Automation is taking over the field at a rapid pace and well captured.
Thank you! I’m delighted that the compilation provided clarity on the historical context and future trends. Indeed, the rapid automation in this field is a fascinating development.